分类 - 图像篡改检测与定位
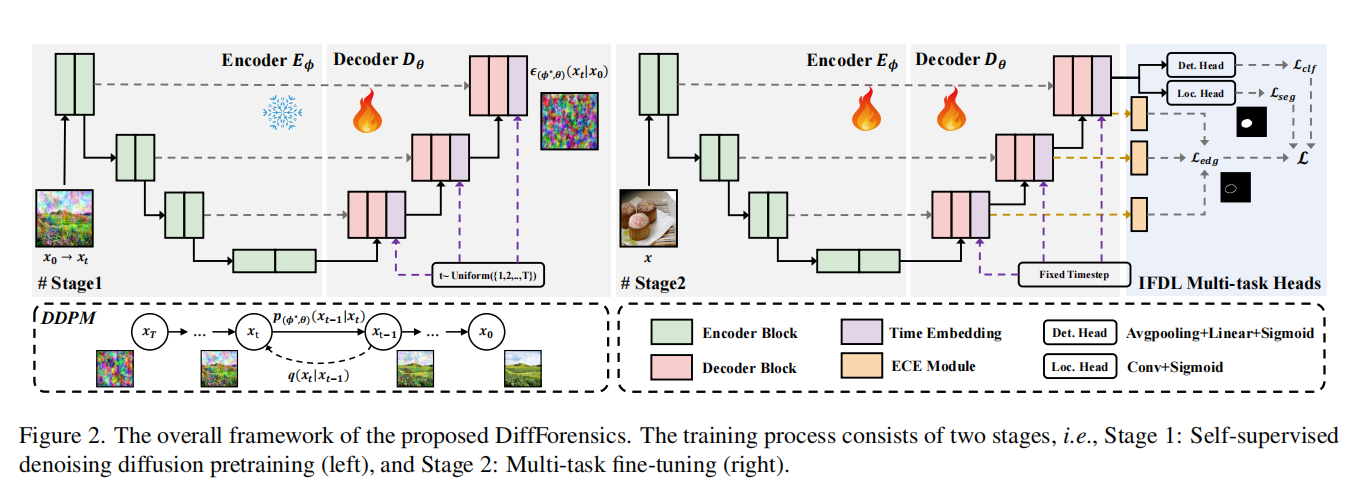
DiffForensics
发表于CVPR2024,两阶段的训练过程,该框架包括自监督去噪扩散的训练前阶段和多任务微调阶段,提出了一种新的边缘提示增强模块,该模块集成在多个尺度的解码器中,以增强被篡改的边缘痕迹从粗到细。
1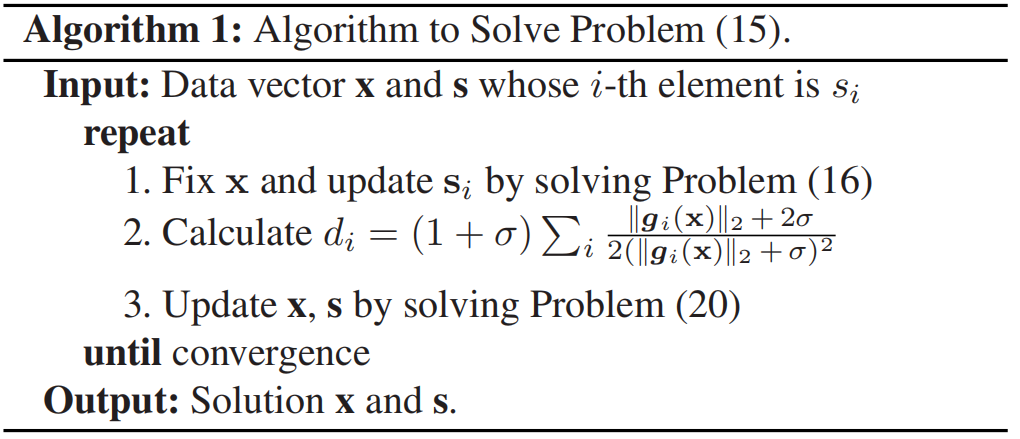
Deep Fuzzy K-Means With Adaptive Loss and Entropy Regularization
发表于IEEE Transactions on Fuzzy Systems 2019,提出了深度模糊k-means(DFKM),具有加权自适应损失函数的FKM。
5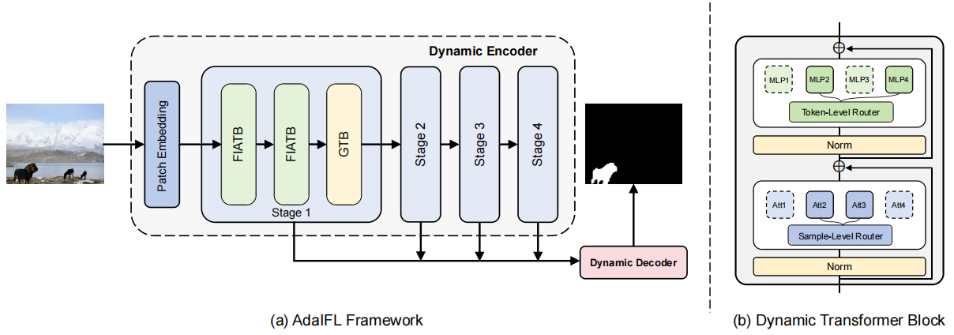
AdaIFL:Adaptive Image Forgery Localization via a Dynamic and Importance-aware Transformer Network
发表于ECCV2024,提出了AdaIFL,为不同的网络组件定制不同的专家组,构建多个不同的特征子空间,利用自适应激活的专家网络,AdaIFL可以捕获与伪造模式相关的判别特征,增强了模型的泛化能力。提出了一种特征重要性感知注意力,自适应地感知不同区域的重要性,并将区域特征聚集成可变长度的标记,将模型的注意力导向更有区别和信息的区域。
6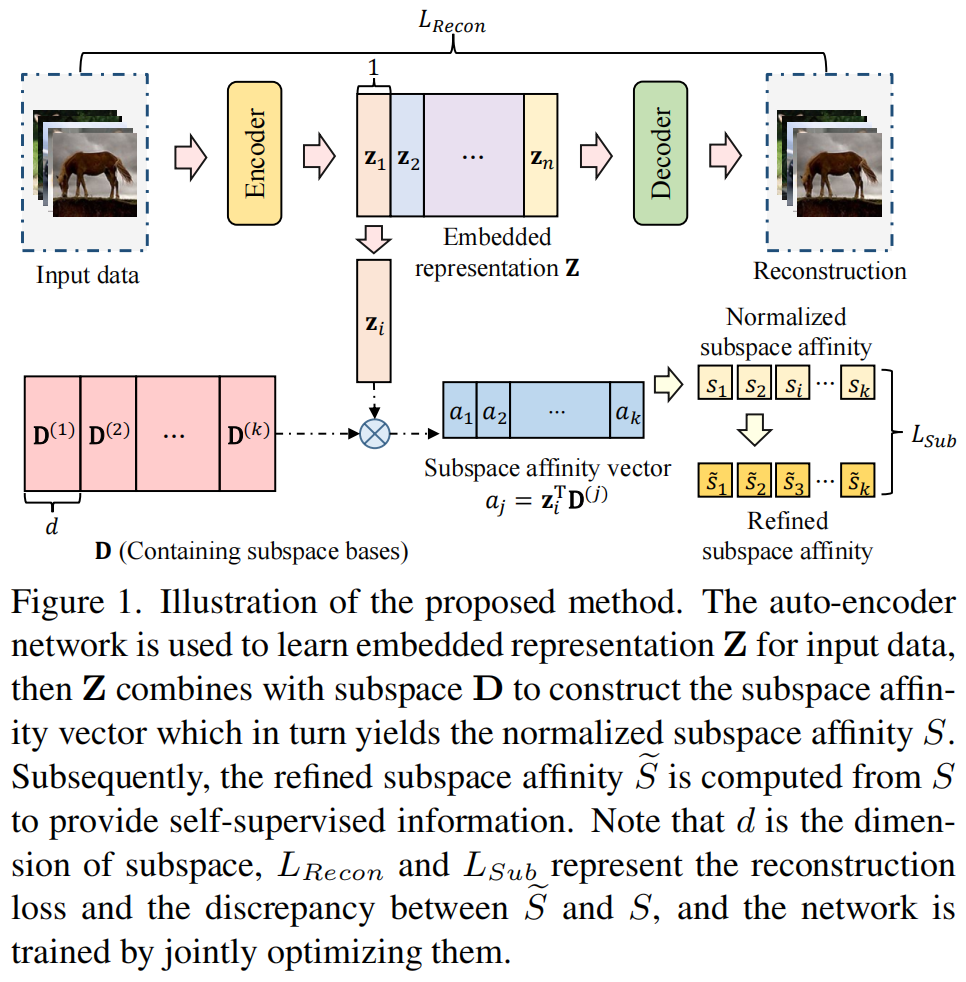
Image_Manipulation_Detection_With_Implicit_Neural_Representation_and_Limited_Supervision
发表于ECCV 2024。
7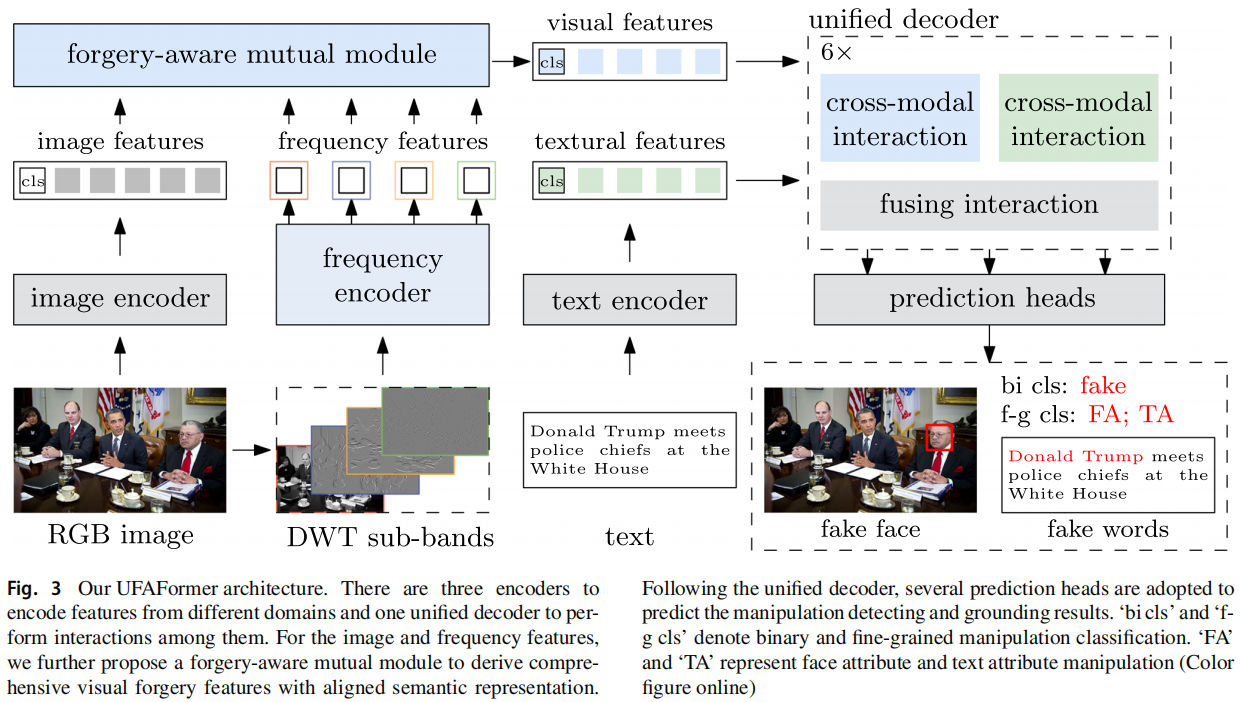
Unified Frequency-Assisted Transformer Framework for Detecting and Grounding Multi-modal Manipulation
发表于IJCV 2024,多模态的图像篡改检测。
8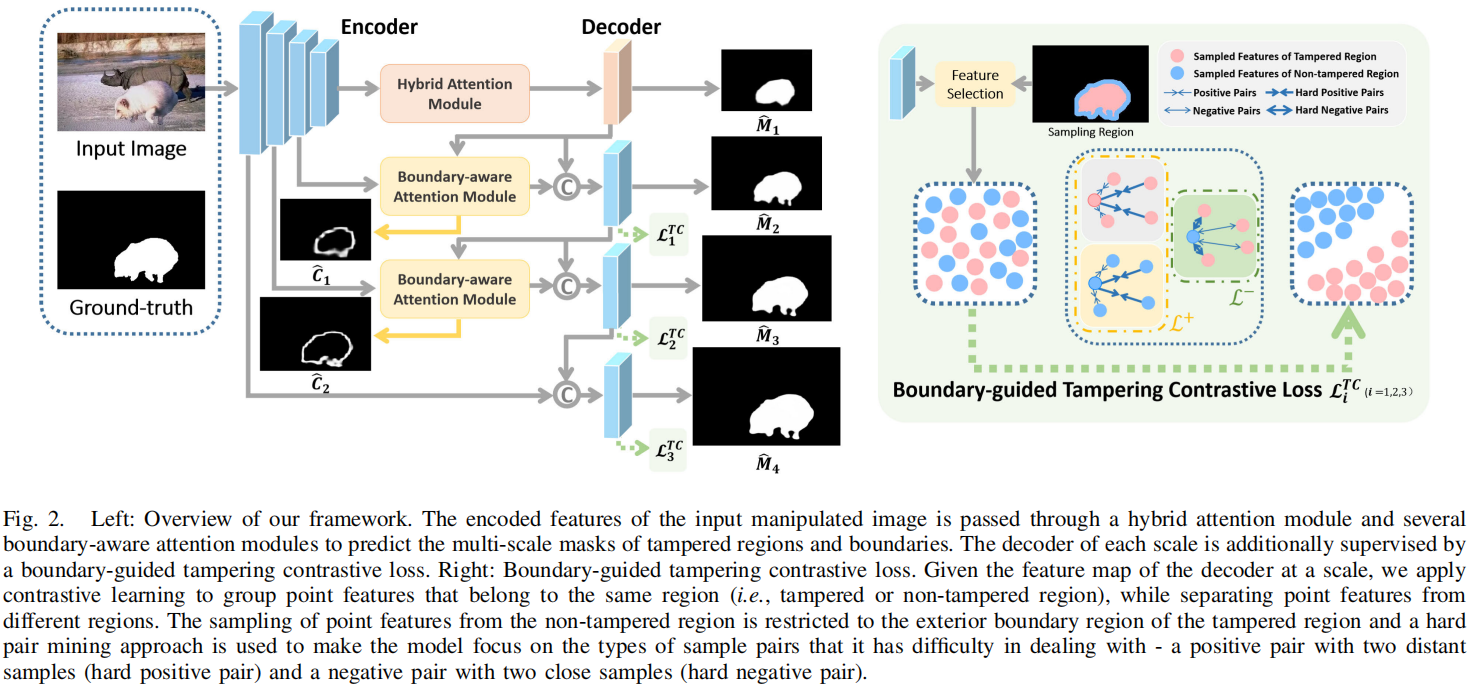
Attentive and Contrastive Image Manipulation Localization With Boundary Guidance
发表于TIFS2024,被篡改区域的边界是分离被篡改和未被篡改像素的关键位置,在这项工作中,我们提出了一种图像操作检测的边界感知方案,其中我们引入了充分利用篡改区域的边界信息,并从注意和特征学习两个角度实现了我们的方案。
*现有问题*: 被篡改区域的边界是分离被篡改和未被篡改像素的关键位置。然而,如何利用这些边界信息来提高检测被篡改图像区域的性能仍有待探索。 *解决方案*: 在这项工作中,我们提出了一种图像操作检测的边界感知方案,其中我们引入了充分利用篡改区域的边界信息,并从注意和特征学习两个角度实现了我们的方案。首先,为了进一步增强操作定位,我们鼓励该模型关注一个被篡改区域周围的边界,其中经常存在非自然的混合。其次,受对比学习的启发,我们寻求学习一个特征空间,即篡改区域内的点远离篡改区域边界附近的非调和区域点,以获得更强大的特性来定位篡改区域。