文章总览 - 114
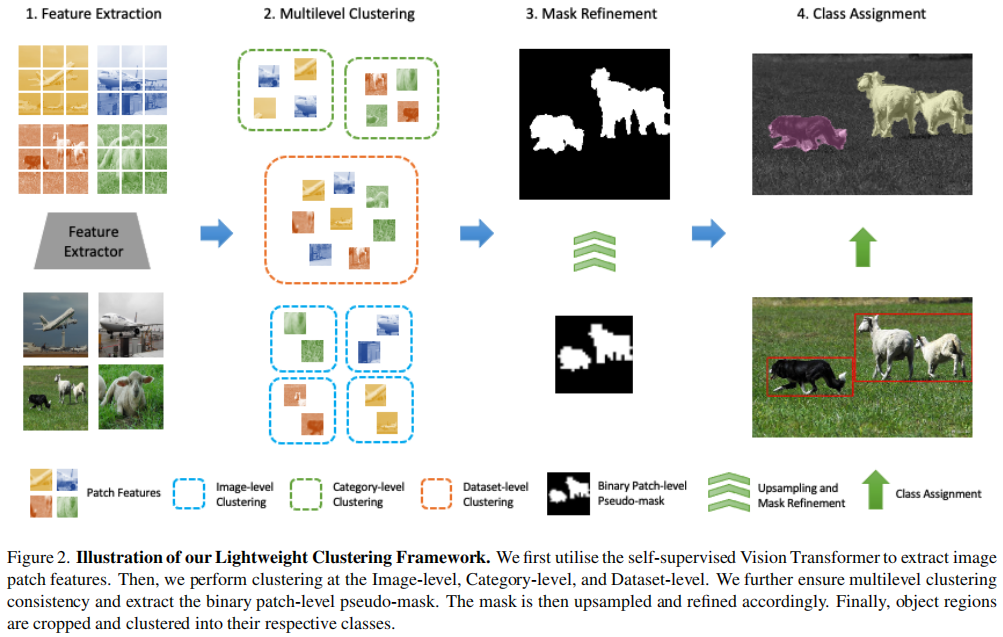
A Lightweight Clustering Framework for Unsupervised Semantic Segmentation
aixiv文章,面对无监督语义分割,使用多级聚类的方法来实现。
21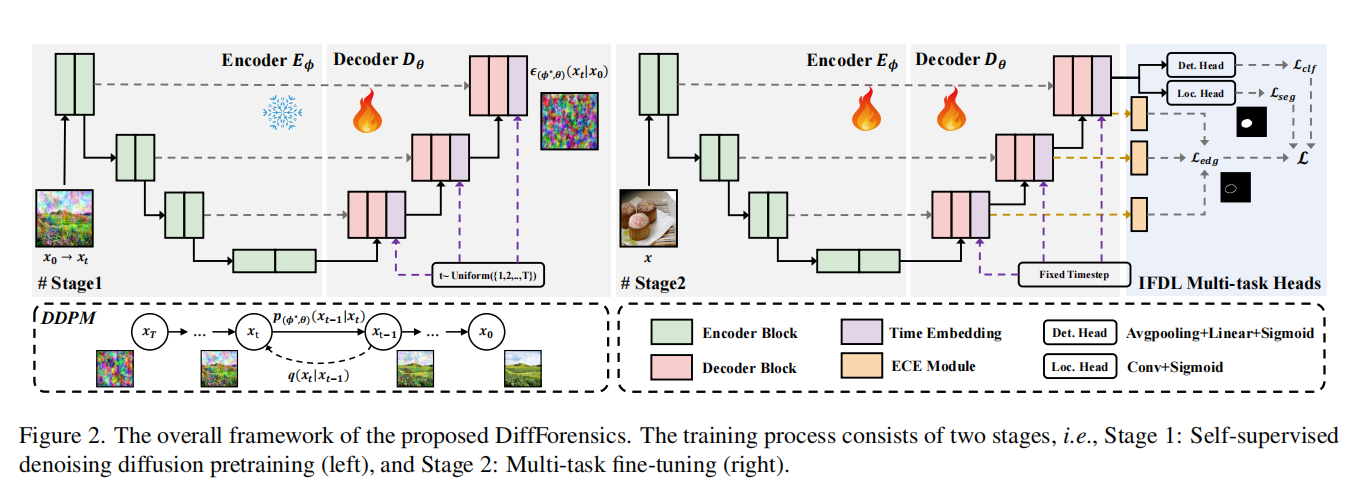
DiffForensics:Leveraging Diffusion Prior to Image Forgery Detection and Localization
发表于CVPR2024,两阶段的训练过程,该框架包括自监督去噪扩散的训练前阶段和多任务微调阶段,提出了一种新的边缘提示增强模块,该模块集成在多个尺度的解码器中,以增强被篡改的边缘痕迹从粗到细。
26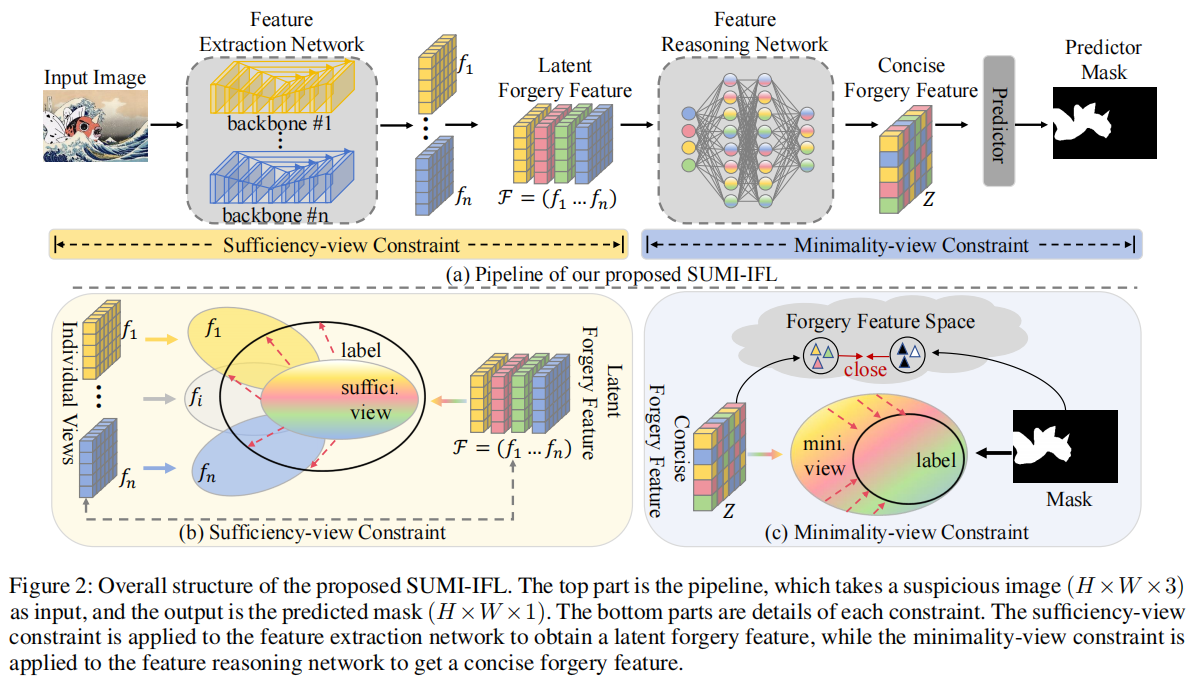
SUMI-IFL:An Information-Theoretic Framework for Image Forgery Localization with Sufficiency and Minimality Constraints
发表于aixiv,使用信息瓶颈理论完成图像篡改任务,没和NP++、IFL-VIT比较。
27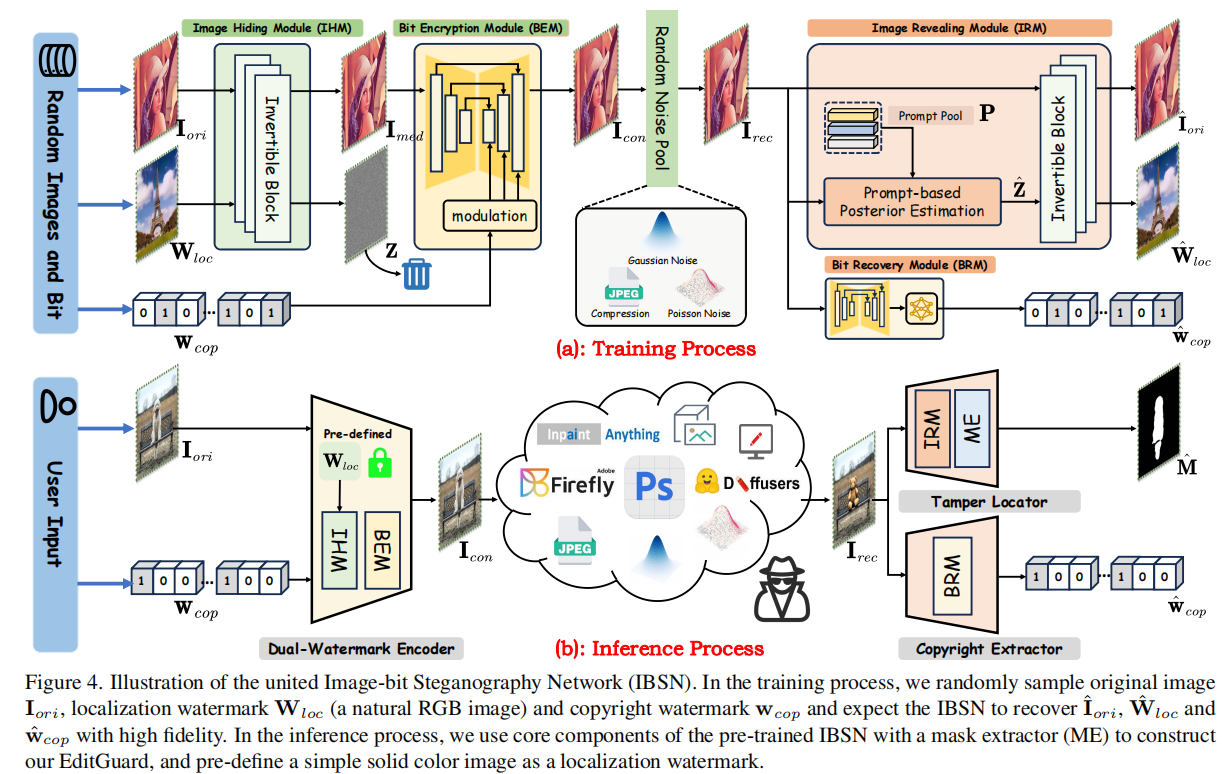
EditGuard:Versatile Image Watermarking for Tamper Localization and Copyright Protection
发表于CVPR2024,将版权水印和图像篡改主动保护两个任务联合起来。
28